Why Traditional Image Quality Specifications Can Be Outdated for Modern AI-Driven Security X-Ray Systems
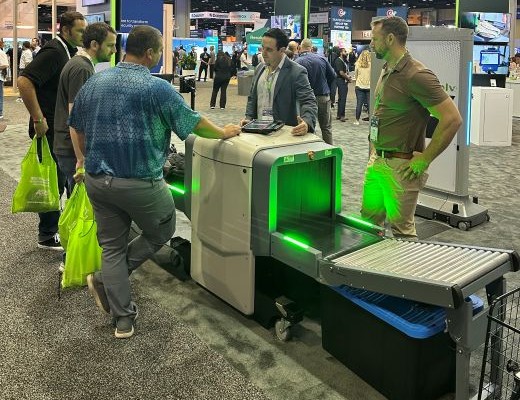
My Background
Before founding Evolv, I founded and led Reveal Imaging Technologies as CEO, where I worked to advance X-ray imaging and automated detection technologies for the aviation security industry. With twenty patents in X-ray inspection and automated detection technology, I bring decades of experience in physical security and threat detection. The article below shares my personal perspective on some of the changes that promise to revolutionize our shared mission.
Executive Summary
Advancements in AI and technology in general are transforming industries, the security industry included. AI enables new capabilities that would have been pipe dreams even 5 years ago, and these capabilities are coming online rapidly. In contrast, the security industry often relies on familiar standards that may not apply directly to the latest technology. New capabilities deserve to be evaluated on their own merits, not necessarily to historical standards.
The evaluation of image quality for security X-ray systems has traditionally relied on specifications developed in an era when human operators were responsible for analyzing images for potential threats. Human perception-based metrics such as resolution, contrast sensitivity, and penetration capabilities have long been the benchmarks for system performance. However, with the advent of modern AI-driven security X-ray systems that utilize sophisticated algorithms to automatically detect threats, these legacy specifications have become increasingly outdated and irrelevant.
This paper argues that the focus of evaluation should shift from traditional human perception based image quality metrics to detection accuracy, processing speed, consistency of detection, and adaptability of AI-based threat detection. Applying the old standards to these new technologies can not only be ineffective but can miss the potential transformative advantages of AI-based automation. It’s like asking for smog test results for a new electric vehicle.
Introduction
Security X-ray systems play a critical role in transportation, public safety, and commercial security by detecting threats such as weapons, explosives, and contraband. Historically, the performance of these systems has been evaluated based on image quality standards, including measures of resolution, contrast, and penetration depth. These specifications were designed with the assumption that human operators would interpret the X-ray images to identify potential threats. However, the introduction of AI-based automated detection systems has fundamentally changed the operational dynamics of security X-ray technology.
The Shift to AI-Driven Threat Detection
AI-based security X-ray systems employ advanced algorithms that are designed to analyze images to identify potential threats automatically. These systems leverage deep learning and computer vision techniques, allowing them to detect a wide range of threat objects with a high degree of accuracy. This shift towards automation is significant for several reasons:
- Efficiency and Speed: AI-based systems can analyze images in a fraction of a second, which creates the potential for high throughput screening in high-traffic environments.
- Consistency: AI systems maintain consistent performance throughout their operation. They do not rely on human operators, who may have various levels of training and competency, and could be impacted by fatigue, distractions, or biases, to interpret images.
- Scalability: These systems can be deployed across numerous checkpoints with minimal variation in performance, enabling standardized threat detection capabilities across different locations.
The Irrelevance of Traditional Image Quality Metrics
The traditional specifications for evaluating X-ray system image quality were developed to ensure that images provided to human operators were clear, detailed, and interpretable. Metrics like resolution and contrast sensitivity were crucial when the task of threat identification relied on the human eye. However, in the context of AI-based automated detection, these specifications lose their significance. Key reasons for this include:
- Automated Analysis Over Human Interpretation: Modern AI systems do not present images to an operator for analysis. Instead, they process the images directly and make decisions regarding the presence or absence of threats. Since the AI is not reliant on visual interpretation, the emphasis on image clarity for human review is no longer relevant.
- Focus on Data Quality Over Image Quality: AI systems require data that is representative of various threat types and scenarios for training purposes. The quality and breadth of this data, rather than the traditional image quality metrics, determines the system’s ability to recognize threats.
- Adaptability to Variable Image Quality: AI algorithms can be trained to compensate for variations in image quality that might hinder human interpretation, such as noise or low resolution, making older standards less applicable.
Analogy: Smog Tests for Electric Vehicles
To illustrate the mismatch between traditional image quality specifications and modern AI-based systems, consider the analogy of smog testing for electric vehicles (EVs). Smog tests are designed to measure emissions from internal combustion engines, ensuring that vehicles comply with environmental regulations. Applying these tests to EVs, which produce zero tailpipe emissions, would be nonsensical. The standards developed for one type of technology simply do not translate to another.
Similarly, using traditional image quality specifications to evaluate AI-based security X-ray systems is not relevant. The AI-based systems do not rely on image quality in the same way as traditional systems because they do not present the image to a human operator. Instead, their effectiveness is better evaluated by the accuracy and consistency of their threat detection capabilities. Applying outdated metrics not only fails to capture the potential advantages of the newer technology but also creates unnecessary hurdles that do not improve security outcomes.
The New Metrics for Evaluating AI-Based X-Ray Systems
To properly assess the performance of AI-based security X-ray systems, the focus should shift to new evaluation criteria that reflect the capabilities and potential advantages of automated analysis. Relevant metrics include:
- Detection Accuracy: The rate at which the AI correctly identifies both threats and non-threats, including detection rates for false positives and false negatives.
- Processing Speed: The time taken by the AI to analyze images and make decisions, which impacts operational efficiency.
- Consistency of Detection: The ability of the AI system to maintain performance levels over time and across different environments.
- Adaptability: How well the AI system can be updated or trained to detect new types of threats as they emerge.
These metrics more accurately reflect the performance of modern security X-ray systems, providing a better understanding of their capabilities and effectiveness in real-world applications.
Conclusion
The rise of AI-based security X-ray systems represents a transformative shift in the field of threat detection, which may ultimately make traditional image quality specifications obsolete. Applying outdated evaluation criteria to these advanced systems can not only be ineffective but can miss the opportunity to leverage the full potential of AI-driven analysis.
By recognizing the need for updated standards—focused on the capabilities of AI rather than legacy metrics—we can help ensure that security X-ray systems are assessed in a way that reflects their true value. This approach aligns with the evolving technological landscape and works to ensure that security measures remain robust, efficient, and effective in meeting modern challenges.
*This paper expresses the opinion of Mike Ellenbogen, a member of the Evolv Technology Executive Leadership Team, about the future of AI-Driven security x-ray systems.